The lecture focused on the analysis of the approximation properties of convolutional neural networks, in relation to the a priori information available. To overcome the curse of high dimensionality, networks must exploit strong forms of regularity. In particular, this regularity involves three types of properties : multiscale separability, the existence of symmetry groups and the existence of parsimonious representations. The lecture studied these three forms of regularity, in connection with the architecture of networks, and the a priori information we have about the problem. This concerns various branches of mathematics, including harmonic analysis and group theory. The lecture and seminars also made the link with neurophysiological models of auditory and visual perception.
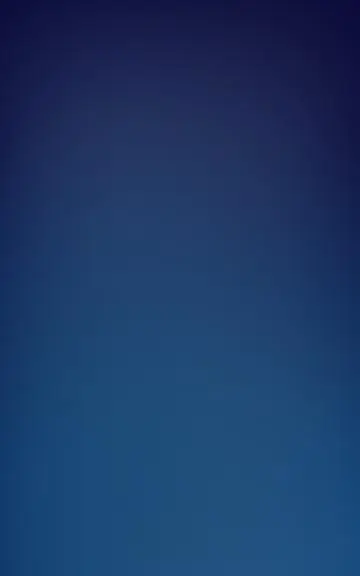
→
Lecture
Multiscale models and convolutional neural networks
→