Résumé
The stunning recent advances in AI content generation rely on cutting-edge, generative deep learning algorithms and architectures trained on massive amounts of text, image, and video data. With different training data, these algorithms and architectures can also be used to confront climate change. As opposed to text and video, the relevant training data includes weather and climate data from observations, reanalyses, and even physical simulations.
As in many massive data applications, creating "labeled data" for supervised machine learning is often costly, time-consuming, or even impossible. Fortuitously, in very large-scale data domains, "self-supervised" machine learning methods are now actually outperforming supervised learning methods. In this lecture, I will survey our lab’s work developing generative and self-supervised machine learning approaches for applications addressing climate change, including downscaling and temporal interpolation of spatiotemporal data and generating probabilistic weather predictions.
Claire Monteleoni
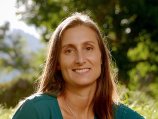
Claire Monteleoni is a Choose France Chair in AI and a Research Director at INRIA Paris, a Professor in the Department of Computer Science at the University of Colorado Boulder (on leave), and the founding Editor in Chief of Environmental Data Science, a Cambridge University Press journal launched in December 2020. Her research on machine learning for the study of climate change helped launch the interdisciplinary field of Climate Informatics. She co-founded the International Conference on Climate Informatics, which will hold its 14th annual event in 2025. She gave an invited tutorial: Climate Change: Challenges for Machine Learning, at NeurIPS 2014. She currently serves on the U.S. National Science Foundation’s Advisory Committee for Environmental Research and Education, and as Tutorials co-Chair for the International Conference on Machine Learning (ICML) 2024 and 2025.